Machine Learning and Data Science
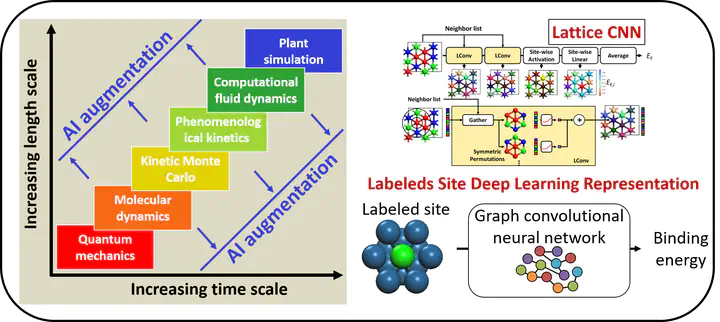
U. S. Materials Genome Initiative’s effort to digitize materials for data science has led to significant materials within a research time frame of a year or two, advancing technology for OLED, drugs, CO2 reduction electrocatalyst, polymers, and Li solid electrolyte to name a few. Notably, machine learning models’ ability to find non-linear correlations between the input and the output has paved new routes to engineering and designing materials. With closing windows of opportunity to limit global climate change, data science shows promises to accelerate to transition to a carbon-neutral society.
Accelerating research
Despite the significant advancement in theory in describing the properties of the materials, some properties are too complex analytically derive or take too much computational resources to simulate. For example, quantum mechanical simulation is computationally expensive. Recent advancement in machine learning potential aims to rapidly predict the energy of the system and predict potential energy surface, thus accelerating quantum mechanical calculations. The reaction mechanism of incredibly complex catalysts is now possible (Gu et al.). The correlations between the larger scale properties can be correlated to smaller scale properties. (Sendek et al. prediction of Li-ion conducting materials.) Bayesian learning framework can be coupled with machine learning to predict the synthesizability of crystals that are otherwise too difficult to quantify (Gu et al.). So-called inverse design approach can be used to generate crystals with desired properties (Kim et al.). Academia has been expanding the prediction capability rapidly, and exciting applications have been innovating the field.
Research focus
We focus on developing innovative, game-changing machine learning models as well as developing databases to perform data-science approaches. We are exploring all fronts of solid state physics and physical chemistry to expand prediction power.